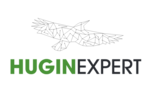
Overview
BayesFraud is a market-leading predictive analytics solution that provides insurers with advanced, automated fraud detection capabilities during claims handling.
Our solution consistently evaluates all submitted claims for fraud and alerts claims handlers to high-risk claims. In this way routine claims can be fast-tracked for immediate settlement, and claims with the highest likelihood of fraud can be investigated further – before costly claims are paid out.
The results: A positive end-to-end claims experience strengthens customer loyalty and retention. And when only high-risk claims are sent to investigators, hit-rate accuracy increases and valuable time and resources can be dedicated to claims with the greatest potential of fraud – and cost savings for insurers.
Key Features
The following table list the features and function the solution support and how it is supported around:
·Data preparation
·Model configuration
·Claims fraud techniques
·Special Unit Investigation (SIU)
Key Benefits
It combines claims data with the incredibly valuable input from claims and fraud experts in one and the same model
It quantifies missing and uncertain data and observations, to “fill in the data holes” in claims data Calculates fraud on the basis of every shred of evidence available
Uncovers insight about fraud patterns and relationships that would otherwise be missed using manual or rules-based processes
Learns from data as more claims data and information become available